Spatiotemporal Analysis for Weak Signals Detection
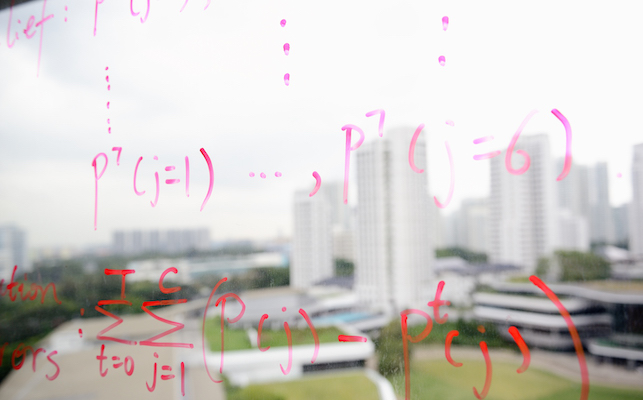
Enhancing sense-making and communication during disruptions through spatiotemporal big data analytics for weak signals detection
Weak signals are unclear observables that could help to predict future states and identify potential disruptions in transportation, community, finance and other systems. However, detecting them in big geospatial data in real time remains a challenge. Current computing frameworks for analysing mobility-related big data are limited because they cannot deal with the massive volume of data, lack real-time capabilities, or do not support context-based analysis.
This research module combines biophysical and cognitive processes to jointly build and improve resilience. Researchers utilise big geospatial data to analyse the flow of people and the underlying physical structures to investigate the sense-making capabilities of users and decision makers of systems. Mobility and transportation in Singapore will be used as a test case since the movement and flows of people could indicate potential disruptions in the near future.
Researchers will develop methods to detect weak signals by integrating spatiotemporal analyses with machine learning, pattern analysis, and geographic information technology, to be applied to big geospatial data obtained from static and mobile sensors. These methods will integrate contextual characteristics based on a semantically rich context. In addition, novel map-adaptation and multimodal interaction mechanisms will be developed in order to effectively and efficiently communicate predicted disruptions and mitigation measures to stakeholders and affected people.
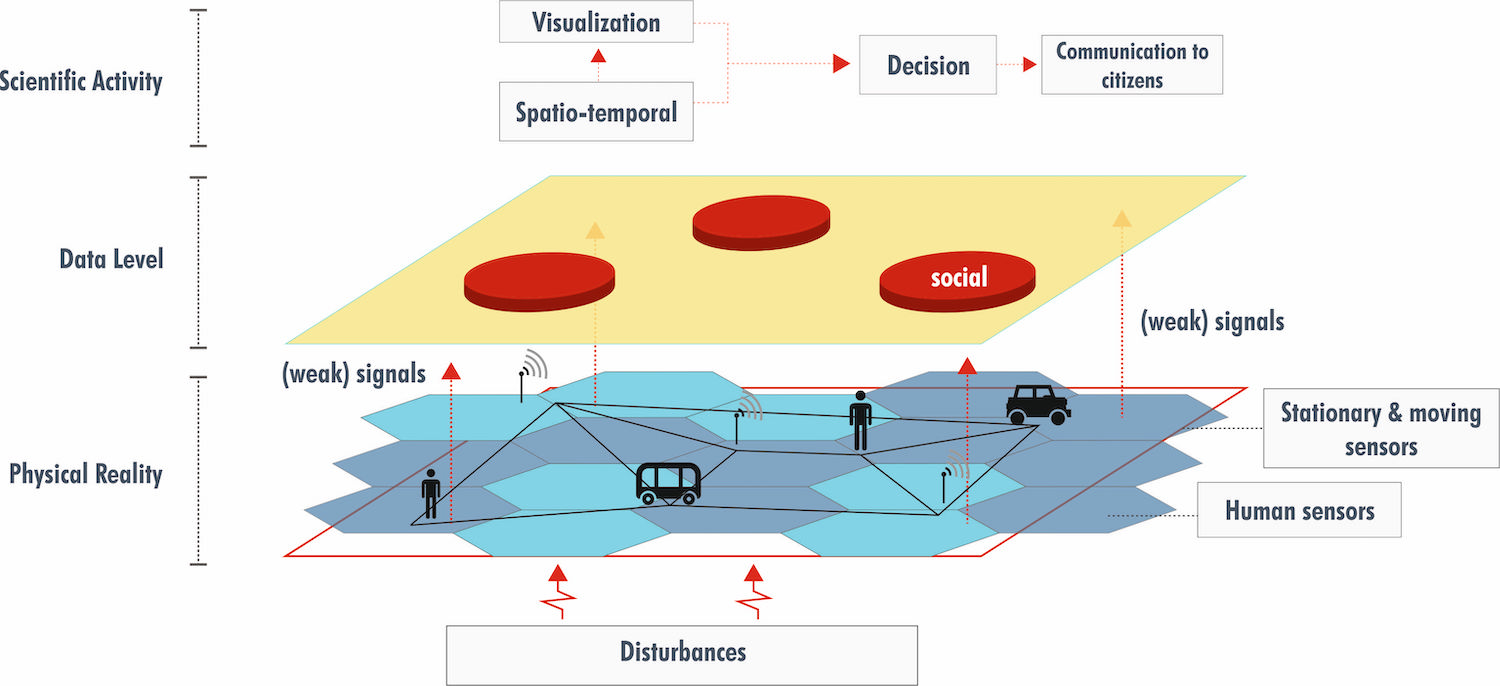
Expected outcomes
The team will:
- Develop a prototype system to detect weak signals in spatiotemporal data under different contexts
- Develop methods for integrating context and handling uncertainty, and an evaluation of how context affects the analysis of mobility data
- Establish a framework for providing notifications and map-based visualisations of predicted disruptions to decision makers and affected individuals. These could include application in collaborative control-room environments and mobile displays, including novel multimodal interaction methods
The methods and specifications developed could contribute to risk and resilience analysis in domains such as transport, ecology, geography, criminology, and human behaviour. Governmental agencies can also use the prototypes developed to monitor various system components of a smart city, detect and forecast potential disruptions, and devise countermeasures.